"Beyond Bias: How Interviews are increasingly missing the Mark on Talent Assessment"
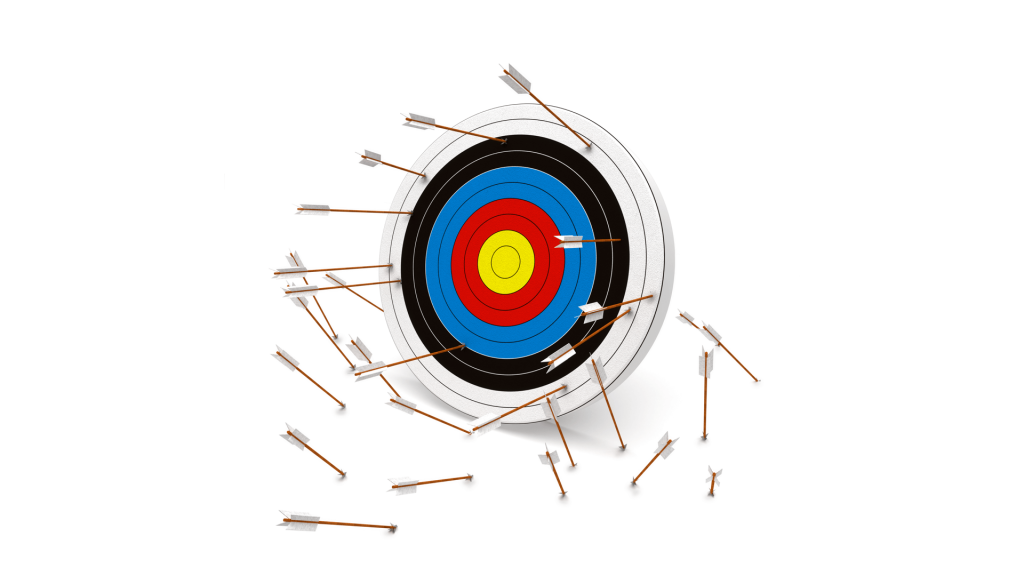
In recent years, the data science field has seen a growing trend: incorporating project-based tasks into the interview process. These assignments often aim to simulate real-world challenges, enabling candidates to showcase their skills in a practical context. On the surface, this practice seems logical and beneficial for both parties. However, as this approach becomes more common, its pitfalls are becoming increasingly apparent. This is something that must be addressed, as candidates waste an incredible amount of time on such tasks.
For candidates, these projects can serve as a window into the organization’s dynamics, revealing its culture, priorities, and understanding of data science. For organizations, however, these tasks often expose deeper issues, including unclear expectations, a lack of strategic vision, and a fundamental misunderstanding of how effective data science operates.
This article explores how interview processes, particularly those centered on project-based assessments, are failing to effectively evaluate talent. It also offers insights into how organizations can refine their practices to foster mutual respect and maximize the value of their interviews as well as their candidates’ time.
The Divide: Clear vs. Ambiguous Interviews
At its core, the effectiveness of an interview hinges on clarity. From the candidate’s perspective, there are two distinct types of interviews:
- The Well-Defined Interview
In this scenario, the organization knows exactly what it needs. The interviewer has a firm grasp of the role’s requirements, the skills needed, and the challenges the candidate will face. Tasks and questions are specific, relevant, and aligned with the organization’s goals. Candidates leave with a clear understanding of what success looks like, and the interview process feels streamlined and productive. The number of steps in the process is clearly outlined, the timeline is set, and a decision deadline is established.
- The Ambiguous Interview
By contrast, many interviews—especially in industries that are new to hiring data specialists—lack this clarity. Organizations often have only a vague idea of what they are looking for. They might know they need “data-driven insights” but struggle to articulate how these insights will be used or what value they will bring. This lack of focus often results in overly broad or poorly designed interview tasks, leaving candidates frustrated and confused. This issue often stems from hiring managers who lack experience in effectively using data, relying instead on superficial metrics or dashboards.
The Project Paradigm: When Projects Go Wrong
One of the most common issues in ambiguous interviews is the use of poorly defined project tasks. For example, a candidate might be given a dataset and asked to answer questions like:
- “How can we improve performance?”
- “Where were we tactically unprepared?”
- “Give us three recommendations to help us win more games.”
On the surface, these questions seem reasonable. However, they are often so broad that they become virtually impossible to answer meaningfully within the interview context.
Why These Tasks Fail
- Misaligned Expectations: The hiring team may have a preconceived “right” answer in mind, often informed by existing staff or management. If the candidate’s analysis doesn’t align with this expectation, they may be unfairly judged, regardless of the quality of their work.
- Lack of Context: Broad questions rarely provide the necessary background or constraints to guide meaningful analysis. Without a clear understanding of the problem, candidates are left to make assumptions, which can lead to misinterpretations.
- Unrealistic Goals: These tasks often assume that these subsets of data alone can reveal actionable insights. Effective data analysis requires collaboration, domain expertise, and a clear understanding of the problem being addressed.
For example, asking a candidate to “identify tactical shortcomings from a season’s worth of match data” assumes that the data itself contains all the answers. In reality, success often hinges on integrating data with contextual knowledge, of which there are many variables.
The other side of the coin:
Some argue that the inherently open-ended nature of data science—its need for extensive cleaning, exploratory modeling, and iterative refinement—justifies the use of similarly broad interview projects. While this is a valid perspective, it raises a critical question: How can candidates be assessed fairly in such a scenario? Presenting a broad, ambiguous task to multiple candidates inevitably leads to highly individual solutions, reflecting unique approaches, assumptions, and interpretations. This variability makes it extraordinarily difficult for interviewers to evaluate candidates on an equal playing field.
Relying on subjective judgment to consolidate these diverse solutions introduces biases and inconsistencies, impeding the ability to fairly compare candidates’ strengths and suitability. A more structured, well-defined project ensures that all candidates operate within the same parameters, allowing for simpler and more equitable evaluation while still testing their problem-solving abilities.
The Role of Open-Ended Exploration in Interviews
While this article critiques the overuse of ambiguous, broad interview tasks, it’s important to reaffirm that open-ended exploration is integral in data science. Diving into new concepts and deriving insights from data in a freeform manner can spark innovation, especially when it arises organically. Often, these explorations are inspired by ideas or practices from other industries, novel methodologies, or even serendipitous connections. This kind of imaginative, unrestricted exploration is central to the creativity that drives groundbreaking work in data science.
How to Incorporate Open-Ended Tasks Fairly
Using open-ended tasks in interviews should be approached with caution. If an organization wishes to include such tasks, they must:
- Be Transparent: Clearly state that the task is exploratory, with no “correct” answers, and that the focus is on understanding the candidate’s unique perspective and process.
- Train Interviewers: Provide training to help interviewers recognize and minimize their biases, ensuring that they assess candidates on the merit of their approach rather than how closely it aligns with preconceived notions.
- Encourage Individuality: Use the exercise to learn from candidates rather than test them. This can provide fresh ideas for the organization while showcasing the candidate's creativity and problem-solving skills.
When done poorly, open-ended tasks can alienate candidates, lead to unfair evaluations, and ultimately hinder the organization’s ability to attract innovative thinkers. When done thoughtfully, however, they can be a valuable tool for uncovering new perspectives and identifying candidates who thrive in creative, unstructured environments.
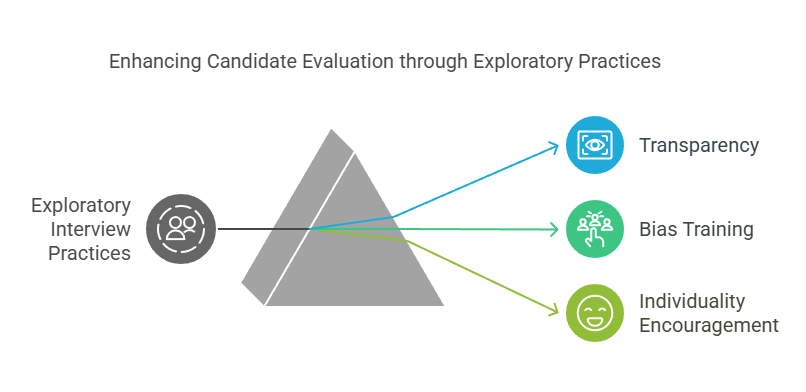
Red Flags in the Interview Process
The Rise of Black Box Obsession
With the increasing prominence of AI ,machine learning and LLM, many organizations have developed what can be described as “black box obsession.” This refers to the belief that advanced algorithms or proprietary systems can solve complex problems without human intervention. This mindset is particularly prevalent in organizations that outsource their data initiatives to large multinational corporations. These companies promise groundbreaking insights but often deliver only superficial or generic solutions.
This obsession is a red flag for candidates. Organizations overly reliant on black box systems often lack the internal expertise to develop actionable, context-aware insights. Candidates should assess whether an organization values internal development and creativity or simply views data science as a plug-and-play solution. Teams that build and manage their own data solutions foster stronger ownership, engagement, and alignment with organizational goals, ultimately driving better results.
The Curse of Dimensionality and Unrealistic Expectations
Another red flag is when an interview project or question is far too broad to be addressed meaningfully with the provided dataset. Candidates are often tasked with open-ended challenges, such as identifying strategic weaknesses or making far-reaching predictions, using incomplete or poorly contextualized data. This is compounded by a pervasive misconception that “more data” automatically leads to better results. In reality, the curse of dimensionality means that as variables increase, the amount of data required to produce reliable insights grows exponentially, often drowning meaningful patterns in noise.
Organizations that fail to recognize these limitations may unintentionally set candidates up for failure, revealing a lack of understanding about the realities of data science.
The Disconnect Between Management and Data Science
A persistent issue in data science hiring is the disconnect between senior management and the realities of how data is effectively used in sports. Hiring managers with limited exposure to data science may assign tasks or ask questions that reflect misconceptions, such as expecting definitive answers to complex questions or undervaluing the iterative nature of data-driven work. For candidates, this is often a red flag that the organization may struggle to integrate data science effectively into its operations.
Another significant red flag to consider during the interview process is the lack of clear role definitions, especially in teams that have just been formed. For positions labeled as data scientist or data engineer, ambiguity around responsibilities—such as whether the role is part-time or full-time—can create confusion and signal deeper organizational issues. Candidates should be cautious when encountering such vagueness, as it may indicate an organization that lacks a coherent vision for its data department.
While it’s understandable that roles may evolve, particularly in the early stages of a department’s formation, this should be communicated transparently. If a role is expected to develop over time, it is vital that the organization clearly outlines its expectations and provides an opportunity for practitioners to shape the role using their expertise. However, the absence of clarity or acknowledgment of this dynamic nature can be a red flag, pointing to potential disorganization or a lack of strategic direction within the team
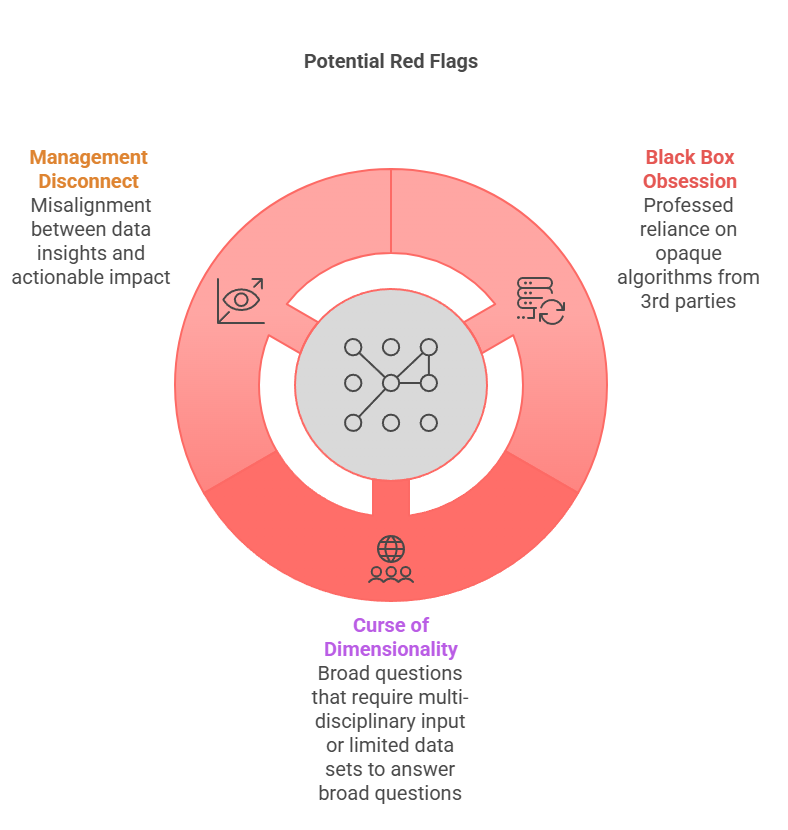
Framework for Improving the Interview Process
To create an effective and fair interview process, organizations must adopt a structured framework:
- Define Problems Clearly: Assign specific, actionable problems rather than vague hypotheticals. Share relevant data openly and avoid ring-fencing critical information.
- Provide Context and Constraints: Offer sufficient background to guide candidates, ensuring they can approach the task with a realistic perspective.
- Encourage Portfolios of Work: Allow candidates to showcase their expertise through past projects, reducing the need for extensive unpaid work.
- Educate Decision-Makers: Train hiring managers to design fair, unbiased tasks and recognize the limitations of open-ended projects.
- Avoid Black Box Solutions: Prioritize building internal expertise, fostering creativity, and aligning data initiatives with organizational goals.
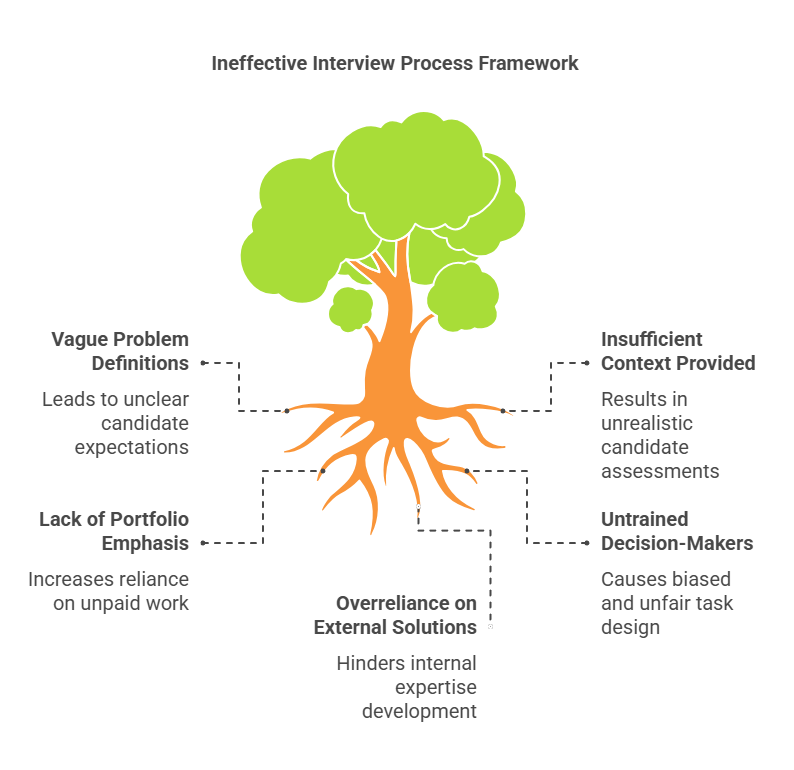
Examples of Good and Poor Interview Task
A good example of an effective interview process I’ve experienced involved a football-related task. The project might ask candidates to build an expected goals (xG) model, with a focus on identifying important variables, feature engineering, and selecting a modelling framework. Candidates would be asked to assess their model, outline its strengths and weaknesses, and present its outputs. To further test practical application, the task might provide a dataset of football players’ shot data and require candidates to identify the most prolific strikers in a particular league based on their xG model or assess the performances of a team in a game and declare who should have won the game based on xG. This approach balances room for unique insights with enough constraints to allow fair comparison across candidates. While there is no single correct answer, solutions can be evaluated on a spectrum of more optimal to less optimal, enabling clear assessment criteria.
In contrast, a poorly crafted, overly open-ended interview might involve providing a season’s worth of data with a huge number variables and asking candidates to answer questions such as, “What were our team’s strengths and weaknesses last season?” or “What should we do next season to ensure success?” Such tasks lack clear guidance or evaluation metrics, making it difficult to compare candidates as unique solutions adds to the dimensionality of evaluation which opens the window to individual biases as to what ‘Good’ looks like and reinforcing group think practices in your department.
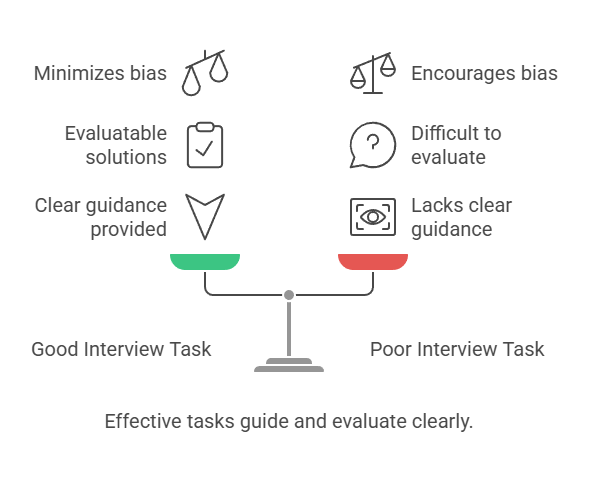
The way an organization approaches interviews speaks volumes about its culture, priorities, and understanding of data science. Misaligned expectations, poorly designed tasks, and unrealistic assumptions risk alienating the very talent these organizations hope to attract. By refining their hiring practices, organizations can create an environment where candidates feel valued and understood, while ensuring that their data initiatives are grounded in reality. In a rapidly evolving field like data science, clarity, collaboration, and creativity are not just desirable—they are essential for success.